Maximizing Business Potential with Image Datasets for Classification
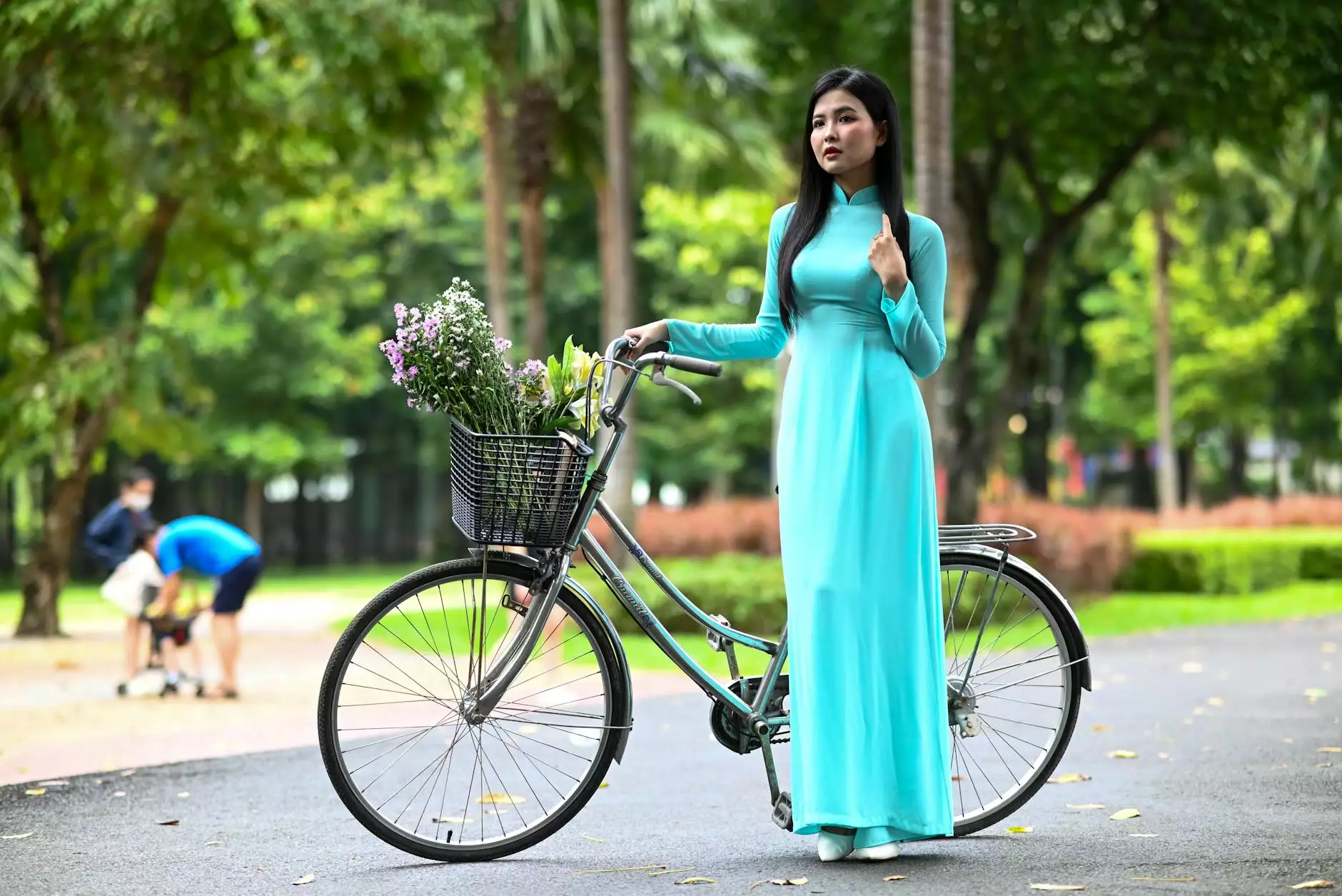
In today’s digital age, businesses are inundated with data. Among various forms of data, image datasets for classification play a pivotal role in driving innovation, improving efficiency, and ultimately enhancing profitability. With the increasing reliance on artificial intelligence (AI) and machine learning (ML), the ability to accurately and efficiently classify images is becoming indispensable.
The Importance of Image Datasets in Business
Understanding image datasets for classification requires a perspective on how they influence business operations. Here are several reasons why improving image classification systems is crucial for modern businesses:
- Enhanced Decision-Making: Image datasets enable businesses to make informed decisions based on data analysis.
- Increased Automation: Automating image classification can reduce manual labor and save valuable time.
- Cost Efficiency: Streamlined processes through well-analyzed data help in reducing operational costs.
- Customer Satisfaction: Accurate classifications lead to better service delivery and customer satisfaction.
Understanding Image Datasets for Classification
Image datasets for classification are collections of images categorized into distinct groups. These datasets serve as the foundational elements for training AI models, enabling machines to recognize patterns and make predictions. Businesses that utilize these datasets can significantly enhance their image recognition capabilities, allowing for a broad range of applications, from security systems to retail analytics.
Types of Image Datasets
Understanding the various types of image datasets is essential for harnessing their potential effectively. Here are the main types:
- Public Datasets: Accessible to everyone, these are usually maintained by educational or research institutions.
- Proprietary Datasets: Owned and managed by businesses or organizations, these datasets are tailored to specific operational needs.
- Custom Datasets: Created to meet unique requirements, they involve intensive data annotation processes.
Key Features of A Good Dataset for Classification
When considering image datasets for classification, it's vital to focus on their characteristics. Here are essential features to look for:
- Size: A large dataset enables more robust training and generalization of models.
- Diversity: Include images from various angles, lighting conditions, and contexts to improve model accuracy.
- Quality: High-resolution images with clear labels lead to better classification performance.
- Balanced Classes: Ensure that each category is well-represented to prevent bias in the model.
Data Annotation: A Critical Component of Image Classification
Data annotation refers to the process of labeling data for training machine learning models. For image datasets for classification, it involves tagging images with relevant labels that indicate what they depict.
The Role of Data Annotation Tools and Platforms
Utilizing a reliable data annotation tool is crucial for efficient data preparation. A top-tier data annotation platform can streamline the annotation process, improving the speed and quality of dataset creation.
Keylabs.ai offers advanced tools designed for high-quality data annotation. Here are some key features that set them apart:
- User-Friendly Interface: Easy-to-navigate tools enhance productivity and reduce onboarding time.
- Quality Assurance: Embedded QA processes ensure that annotations are accurate and consistent.
- Scalability: Capable of handling projects of varying sizes, making it suitable for both startups and large corporations.
Implementing Image Datasets for Classification in Business
Integrating image datasets for classification into your business strategy involves several steps:
1. Define Your Goals
Identify what you seek to achieve with image classification—be it improving customer service, enhancing security systems, or gaining insights from retail analytics.
2. Collect Data
Gather images relevant to your goals. Utilize both public and proprietary datasets while ensuring diversity in participants.
3. Annotate the Data
Use an efficient data annotation tool like Keylabs.ai to categorize the images accurately. Annotation quality significantly impacts model performance.
4. Train Your Model
Input your annotated dataset into machine learning models that fit your objectives, optimizing them to recognize and classify images effectively.
5. Evaluate Performance
Monitor the model's success in classifying images. Adjust the dataset and retrain the model as necessary to increase accuracy.
Challenges in Using Image Datasets
While using image datasets for classification can bring numerous benefits, there are challenges that businesses should prepare for:
- Data Privacy: Ensure compliance with regulations to protect sensitive information.
- Quality Control: Maintaining high standards in data annotation requires a systematic approach.
- Model Bias: Be wary of model bias stemming from an imbalanced dataset that underrepresents certain classes.
Future Trends in Image Classification
The future of image datasets for classification is promising, with emerging trends shaping how businesses approach data. Here are some anticipated developments:
1. Use of Synthetic Data
As data privacy concerns rise, synthetic data generation may become more prevalent, allowing businesses to create realistic datasets without compromising privacy.
2. Increased Automation
Automated data annotation processes will continue to evolve, reducing human involvement while maintaining accuracy.
3. Multi-Modal Datasets
Integrating datasets containing various types of data (e.g., images and text) will enhance the understanding and training capacity of AI models.
Conclusion
The integration of image datasets for classification into business strategies is not merely an option but a necessity in today’s competitive marketplace. By utilizing robust data annotation tools and platforms like Keylabs.ai, companies can optimize their image classification processes, leading to improved decision-making and operational efficiency.
As we continue to navigate the future of technology and data, understanding and implementing these strategies will position businesses at the forefront of innovation and success.